Not completely fair, but maybe fair enough for games.
"Researchers have figured out how to design dice with even more exotic shapes, like a kitten, a dragon, or an armadillo. And they are "fair" dice: Experiments with 3D-printed versions produced results that closely matched predicted random outcomes, according to a forthcoming paper currently in press at the journal ACM Transactions on Graphics."
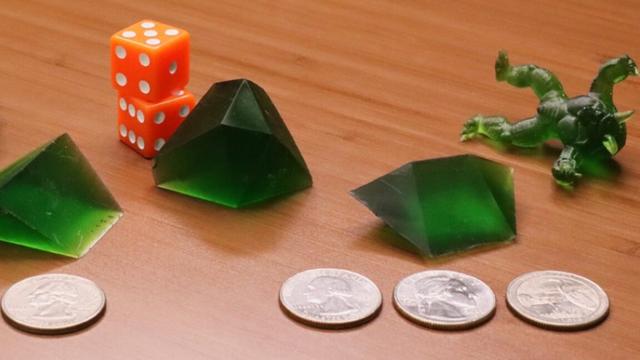